

What Are The Main Types of Artificial Intelligence (AI)?
Artificial Intelligence (AI) refers to machines or software displaying cognitive abilities that mimic human intelligence – such as learning, reasoning, and problem-solving. In business and technology, AI has become a cornerstone of innovation, driving automation, efficiency, and new opportunities. From optimizing manufacturing lines to forecasting supply chain needs, AI is transformative. Its significance is evident in the numbers: for example, AI could contribute up to $15.7 trillion to the global economy in 2030, and worldwide spending on AI technologies is projected to reach $337 billion by 2025. Companies across industries are rapidly adopting AI; in fact, 78% of organizations have implemented AI in at least one business function. Such trends underscore why decision-makers are keen to understand the types of AI and how they can be leveraged for business advantage.
But what are the main types of AI? Depending on whom you ask, you might hear different answers to “how many types of AI are there.” The key is that experts classify AI in two fundamental ways – by capability and by functionality. By combining these, one can outline seven types of AI in total, covering everything from simple artificial intelligence systems to hypothetical future machines. This comprehensive overview will explain each category in clear terms, with industry-relevant examples in manufacturing, supply chain, logistics, and more. Understanding these AI types will help leaders grasp what’s possible today and what may emerge tomorrow.
AI in Business: A Brief Definition and Significance
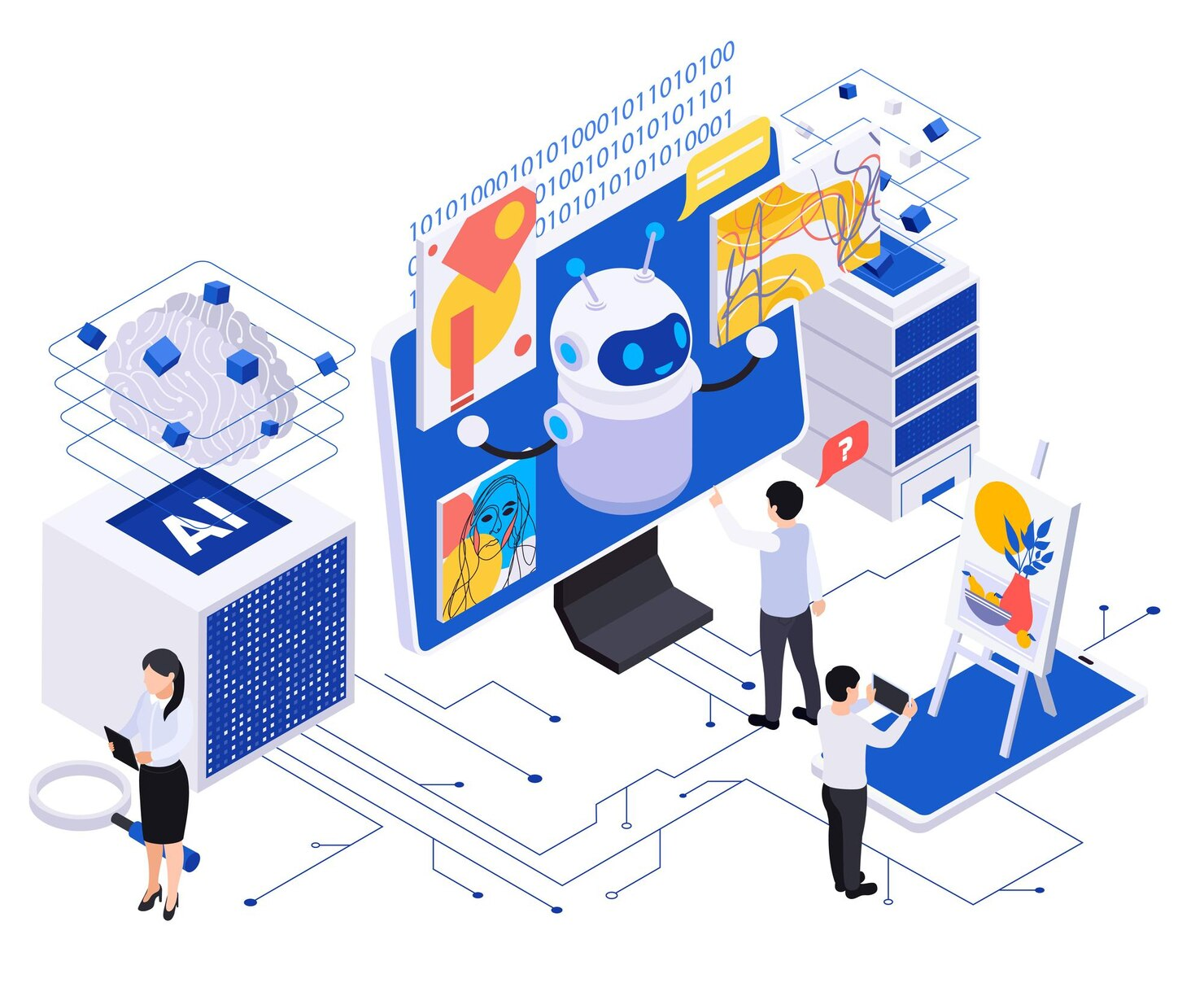
Source: Freepik
Before diving into classifications, let’s define AI in a business context. Artificial Intelligence is essentially software (or machines) that can perform tasks which normally require human intelligence. This includes learning from data (often via machine learning), recognizing patterns (like detecting defects on an assembly line), making decisions, or even conversing in natural language. Unlike traditional software that follows explicit instructions, AI systems can improve over time through experience or additional data.
AI’s significance in business and technology lies in its ability to “learn” and adapt, unlocking levels of efficiency and insight that static programs cannot match. We are already seeing AI’s impact across sectors. In manufacturing, AI-driven predictive maintenance helps prevent equipment failures, and AI “Lighthouse” factories have demonstrated rapid improvements in productivity and quality. In logistics and retail supply chains, AI optimizes delivery routes and inventory levels, cutting costs and response times. A survey by McKinsey found that manufacturing and supply chain are the top functions seeing cost savings from AI – 64% of respondents saw reductions in manufacturing costs and 61% in supply chain planning. In one example, Procter & Gamble’s use of AI and IoT to automate warehouses delivered $1 billion in annual savings. Clearly, AI is not just a buzzword; it’s a practical tool delivering real ROI. Gartner analysts note that the main benefits of AI adoption include increased efficiency and better forecasting (leading to cost reductions) rather than just cutting labor.
Crucially, all these current applications of AI – from a simple recommendation engine to a self-driving forklift – fall under what we call Narrow AI. To better understand AI’s present and future, let’s explore the two primary ways to categorize AI.
Capability-Based Types of AI
The first classification is capability-based, delineating AI by the breadth and generality of its intelligence. In this system, there are three main types of AI:
- Artificial Narrow Intelligence (ANI) – also known as Narrow AI or Weak AI.
- Artificial General Intelligence (AGI) – sometimes called General AI or Strong AI.
- Artificial Superintelligence (ASI) – a theoretical level beyond human intelligence.
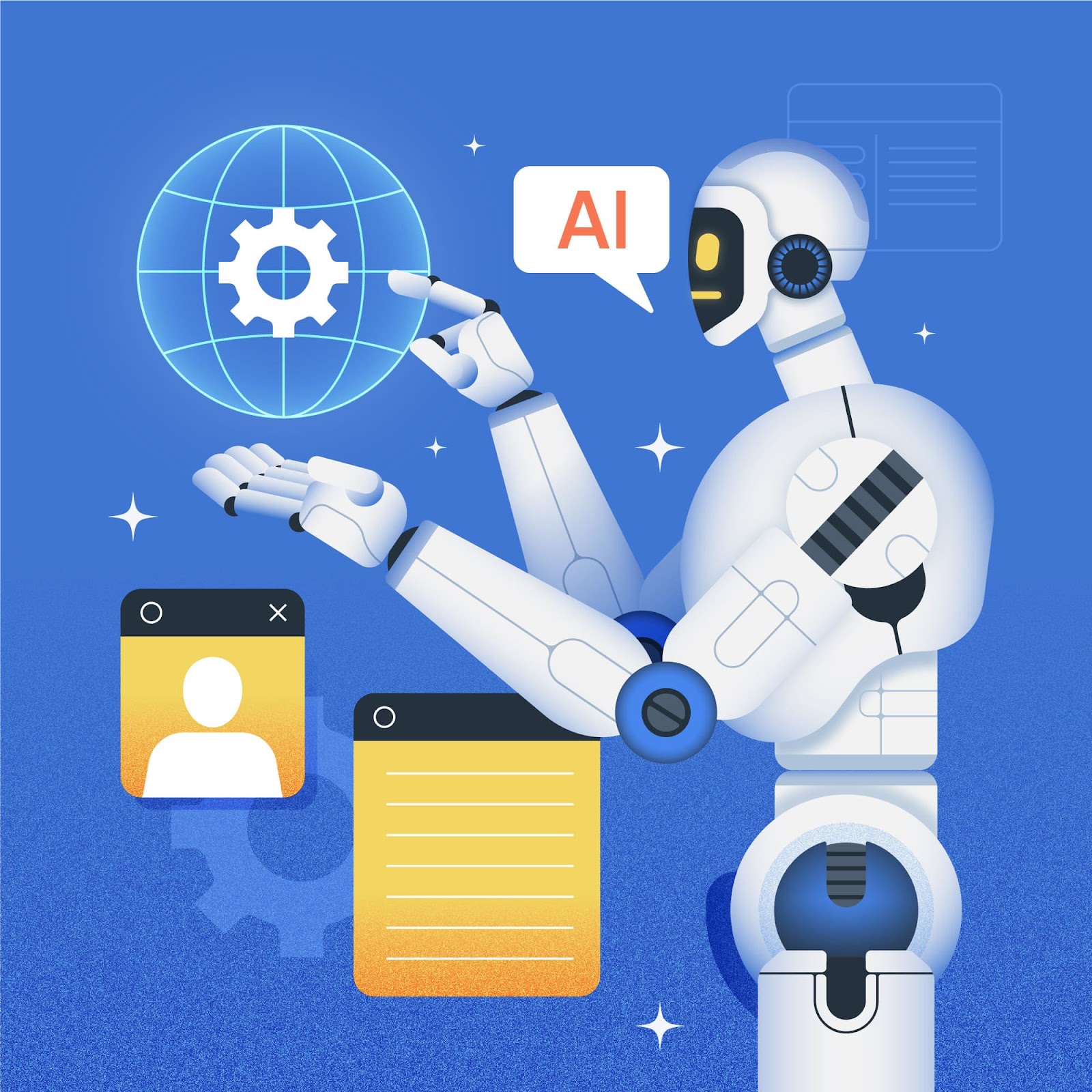
Source: Freepik
1. Artificial Narrow Intelligence (ANI) – “Narrow AI”
Narrow AI refers to AI systems that are highly specialized at a specific task or a narrow domain. These AI types excel in the particular function they are designed for, but cannot generalize their competence beyond those tasks. Nearly all AI in use today is ANI – from customer-service chatbots to advanced industrial robots – and this is where businesses are reaping benefits now.
Common examples of Narrow AI include image recognition software, language translation tools, recommendation algorithms, and many industrial automation systems. In manufacturing, an AI-powered quality inspection camera that detects product defects on a production line is a classic Narrow AI: it performs one job (vision inspection) with superhuman speed and accuracy, but it cannot, say, manage your inventory or drive a vehicle. Likewise, in supply chain operations, AI forecasting models that predict demand or route optimization algorithms that adjust delivery schedules are powerful but single-focused intelligence.
Narrow AI’s impact on business is significant – for instance, an AI system can sift through thousands of supply-and-demand variables to optimize a logistics network far faster than any individual. Operators that applied Narrow AI in industrial processing plants reported a 10–15% increase in production and 4–5% increase in profits (EBITDA) – a substantial performance boost. These “simple” artificial intelligence solutions are the workhorses of the current AI era, proving their value in real-world operations every day.
Operators that applied Narrow AI in industrial processing plants reported a 10–15% increase in production
It’s important to recognize that narrow AI doesn’t truly “understand” or reason generally – it lacks broader awareness. As IBM succinctly explains, today’s AI systems excel at pattern recognition and automation but “lack genuine understanding” and can’t adapt outside their training scope. In other words, they cannot generalize their knowledge to unrelated tasks. This limitation is what separates ANI from the next category, which has long been the holy grail of AI research.
2. Artificial General Intelligence (AGI) – “General AI”
Artificial General Intelligence (AGI) is the hypothetical AI that achieves human-level intelligence across all domains – it can learn and understand any task or topic that a human can. An AGI would be able to generalize learning from one context to another, reason, plan, and intuit across a wide range of subjects. In essence, AGI could perform any intellectual task that a human being can, and likely much faster.
It’s important to note that AGI does not exist today. All current AI achievements are in the realm of Narrow AI. However, AGI is an active area of research and a popular topic in both scientific and business circles because of its disruptive potential. Visionaries in tech – including people like Elon Musk, Sam Altman, and others – have speculated on when AGI might arrive, with some optimistic (or bold) predictions that we could see human-level AI within this decade. There is no consensus on the timeline; many researchers believe we are still decades away, while a few think early forms of AGI could emerge sooner.
What would AGI mean for business? Potentially, everything. An AGI system would not be confined to one task – it could hypothetically design a marketing strategy in the morning, debug software by noon, and formulate a supply chain plan in the afternoon, all while conversing naturally with employees. It would be an AI that understands context and transfers knowledge across domains. For example, an AGI assistant in a wholesale distribution company might observe that a supply chain delay (logistics domain) will impact a marketing promotion (sales domain) and proactively suggest solutions, demonstrating a breadth of understanding like a human executive.
Today, we see early hints of more general AI capabilities with advanced models like GPT-4 or other large language models, which can perform a wide array of tasks (from writing code to answering business questions). Yet, these are still considered advanced Narrow AI, not true AGI, because they lack certain human cognitive abilities like genuine understanding, common-sense reasoning, and autonomous goal-setting beyond their training.
Leading organizations are starting to prepare for an AGI future just in case, by investing in robust data foundations and AI governance. IBM advises that while the timeline for AGI is uncertain, businesses can “prepare… by building a solid data-first infrastructure today”. In practical terms, focusing on strong data practices and integrating Narrow AI solutions now will put companies in a better position to leverage AGI if and when it arrives. Forward-thinking firms (and AI providers like turian) are also emphasizing ethics and safety, knowing that a powerful general AI needs careful oversight to be used responsibly.
In summary, AGI remains mostly theoretical but increasingly less “science fiction” than before. It’s the next frontier that could eventually revolutionize every industry – but for now, it’s an aspiration on the horizon, with Narrow AI doing the heavy lifting in the present.
3. Artificial Superintelligence (ASI) – Beyond Human Intelligence
Artificial Superintelligence (ASI) refers to a level of AI that greatly exceeds human cognitive abilities in virtually all areas. If AGI is human-like intelligence, ASI would be above human intelligence – capable of outperforming the brightest human minds in every field, whether it’s scientific creativity, general wisdom, social skills, or technical prowess. An ASI might not just solve problems faster than any person; it could conceive solutions and innovations that humans couldn’t even imagine.
It’s critical to understand that ASI is entirely hypothetical at this stage. We have no examples of it, and it’s a subject of speculation among futurists and scholars. However, it is frequently discussed in the context of long-term AI implications and ethics. The idea of superintelligence raises profound questions: If a machine becomes far smarter than humans, who controls it? How do we ensure it acts in humanity’s interest? These questions, once the realm of science fiction, are now taken seriously by experts in AI ethics. Thought leaders like Nick Bostrom have warned about the "control problem" – making sure a superintelligent AI’s goals align with ours – and tech luminaries like the late Stephen Hawking and Elon Musk have publicly expressed concern about unchecked superintelligent AI.
In business terms, ASI is not something decision-makers need to plan for in the near future – but it’s worth being aware of as the extreme end of the AI spectrum. It’s the reason why discussions about AI governance and ethical frameworks are happening alongside the more immediate AI deployments. Companies adopting AI today, especially those pushing the envelope (e.g., using autonomous decision-making systems), are encouraged to embed ethics and oversight into their AI projects. Doing so not only prevents current missteps but also paves the way for a safe evolution toward more advanced AI. As a Boston Consulting Group whitepaper noted, rapid advances in AI (like generative AI) are fueling speculation about entering an AGI era, bringing potential risks and ethical considerations that must be addressed. Those considerations multiply manifold when pondering ASI.
In summary, ASI is a theoretical construct – essentially the end-state of AI development where machines surpass human intelligence entirely. It’s a reminder that AI progress, if it continues unabated, could lead to outcomes that are both awe-inspiring and challenging to manage. For now, business leaders should keep ASI in the back of their minds as a philosophical checkpoint and focus on harnessing AI’s proven narrow capabilities, all while supporting responsible AI practices.
Functionality Based Types of AI
The second way to classify the types of AI is by functionality, meaning how the AI operates or learns in relation to human cognitive functions. This framework outlines four types of AI:
- Reactive Machines – AI systems that react to inputs with no memory of past events.
- Limited Memory AI – systems that learn from historical data to inform future decisions.
- Theory of Mind AI – a theoretical category of AI that can understand emotions and mental states.
- Self-Aware AI – the hypothetical AI that has its own consciousness and self-awareness.

Source: Freepik
This classification effectively describes stages of AI sophistication from a cognitive perspective, and it maps loosely to the evolutionary path of AI development. Notably, the first two (Reactive and Limited Memory) exist today, while the latter two (Theory of Mind and Self-Aware) are still theoretical. Let’s look at each in detail with examples and context.
4. Reactive Machines – Rule-Based, No Learning
Reactive Machines are the most basic form of AI systems. A reactive AI follows the simplest form of intelligence: stimulus and response. These systems do not have any memory or data retention capability; they cannot learn from past experiences. Instead, a reactive machine is programmed to respond to a specific set of inputs with a specific set of outputs, always reacting the same way to the same stimuli.
A classic example of a reactive machine is IBM’s Deep Blue, the chess-playing computer that defeated world champion Garry Kasparov in the 1990s. Deep Blue did not “learn” from previous matches in the way modern machine learning does; it simply calculated the best move in reaction to Kasparov’s moves, using predefined algorithms and an enormous database of chess positions. Each turn was treated as a new situation with no memory of prior turns (outside of the immediate game state).
In a business context, we saw many early automation systems with reactive AI characteristics. Early industrial robots and control systems often fell into this category – for instance, a robotic arm on an assembly line that tightens a bolt whenever a car chassis comes into its frame. It performs the action based on a sensor trigger (reacting to “car present” sensor) but has no memory of how many bolts it tightened yesterday or any learning process to improve its speed or precision. In logistics, consider a simple automated sorting machine that redirects packages based on their barcode. It uses if-then rules: if barcode starts with X, send to chute A; if Y, to chute B. It’s essentially a reactive system processing current inputs (the barcode) against a lookup table, without learning. Such systems are reliable and fast for structured tasks, but not adaptable.
Reactive machines can achieve high performance in stable environments. For example, a thermostat is a simple reactive system – it turns the heater on or off in response to the temperature reading, with no need to recall past temperatures. In manufacturing, basic quality control systems might trigger an ejector to remove a defective product when a sensor reads an anomaly – again, a straightforward reaction. These could be considered “simple artificial intelligence” in that they mimic a tiny sliver of intelligent behavior (following rules to make a decision), but they are not intelligent beyond those hard-coded responses.
It’s worth noting that many machine learning models in production act like reactive machines when deployed – they don’t continue learning on the fly, they just apply their learned model to new data inputs to generate an output. For instance, a trained image recognition AI in a factory camera will label defects (output) as it sees them (input), but typically that deployed model isn’t updating itself in real-time with each new image (that would be online learning, which is less common in practice). In that sense, the model’s behavior is reactive: see image -> output label, every time, without self-modification.
In summary, Reactive AI systems:
- Have no memory, no internal learning from past operations.
- Operate on present input only, using predefined rules or learned associations.
- Are suitable for static, repetitive tasks where the rules of the game don’t change over time.
While limited in capability, reactive machines laid the foundation for automation and still serve in many roles where reliability and consistency are paramount. However, as soon as you need the system to improve through experience, you move to the next category: Limited Memory.
5. Limited Memory AI – Learning from the Past
Limited Memory AI includes the majority of AI systems around us today. These are machines that can retain and learn from past data to inform their decisions. The term “limited” memory indicates that there is some memory or training data that the AI uses – essentially, these systems look at historical information and learn patterns to apply to new situations. However, they don’t possess an infinite or long-term memory of every interaction (like a human’s rich memory); their “memory” is typically limited to the data they have been trained on and sometimes transient observations that are explicitly stored.
Machine learning models are prime examples of Limited Memory AI. When a neural network is trained to detect anomalies in a production line, it adjusts its internal parameters based on historical data (e.g., images of good vs. defective products). Once deployed, it uses what it “learned” to evaluate new products. Similarly, an AI system for demand forecasting in a wholesale business will use past sales data, seasonal trends, and maybe recent market indicators to predict next month’s inventory requirements – it is literally using memory (historical data) to make a future decision.
Real-world examples abound:
- Self-driving cars are Limited Memory AI systems. They observe other vehicles’ speed and direction over time, remember traffic light changes, and incorporate map data. A self-driving car must recall, at least for a short time, what nearby cars have been doing (were they braking suddenly a few seconds ago?) to safely navigate. It builds a limited memory representation of the environment – but it’s not storing every trip in perpetuity, just enough context to make immediate driving decisions.
- Manufacturing robots with vision systems often employ limited memory. For instance, a robot welding machine might adjust its alignment based on the last few welds’ data, correcting any drift – it “remembers” recent error measurements to improve the next weld. In quality control, an AI might track the last several products it assessed to calibrate itself (e.g., adjusting sensitivity if it flagged too many false positives in a row). These are ways limited memory appears in industrial AI.
- Chatbots and virtual assistants also use limited memory. Advanced customer service bots will reference earlier parts of a conversation (a short-term memory) to provide contextually relevant answers. However, they don’t truly understand or remember you beyond the current session unless connected to a profile database. Even voice assistants like Alexa use past data (your voice samples, past corrections) to better interpret your commands in the future.
The key difference from reactive machines is that Limited Memory AI improves over time. It has a feedback loop: it can take new data, incorporate it (often by retraining or updating some state), and thus modify its behavior. Most business AI applications – fraud detection systems, recommendation engines, supply chain optimization tools – are limited memory systems that were trained on historical datasets and continue to learn from new data periodically.
However, limited memory AI does not fully understand the “world” or context like a human. It’s not conscious of its learning; it simply updates parameters. Also, its memory is typically task-specific. For example, an AI vision system in a warehouse might remember object appearances, but it has no concept of why those objects matter or any broader situational awareness.
In practical deployment, companies must feed these AI systems with the right historical data. The quality and quantity of training data largely determine how well limited memory AI performs. That’s why data strategy is crucial – as the saying goes, “garbage in, garbage out.” Firms in manufacturing and logistics are consolidating data from sensors, machines, and transactions to give their AI systems richer “memories” to learn from. The reward is substantial: better predictions, fewer errors, and improved efficiency. A Deloitte survey of manufacturers noted that 93% believe AI (predominantly limited memory AI) will be pivotal for driving growth and innovation in their operations – a reflection of industry’s confidence in these learning systems.
To sum up, Limited Memory AI systems:
- Learn from historical data (examples, observations, or experiences).
- Improve their model or decision-making based on this training (and sometimes continued feedback).
- Represent the vast majority of AI applications today – from ML models to complex automation that adapts over time.
They still lack full adaptability outside their trained scope, but they are far more powerful than purely reactive systems. Limited memory AI corresponds to what people often refer to as machine learning or deep learning systems. As such, this category is where most of the current AI business value is generated – whether it’s predictive analytics in supply chain management or dynamic pricing in wholesale distribution, the engine under the hood is a limited memory AI learning from past data.
6. Theory of Mind AI – Understanding Emotions and Intentions (Future AI)
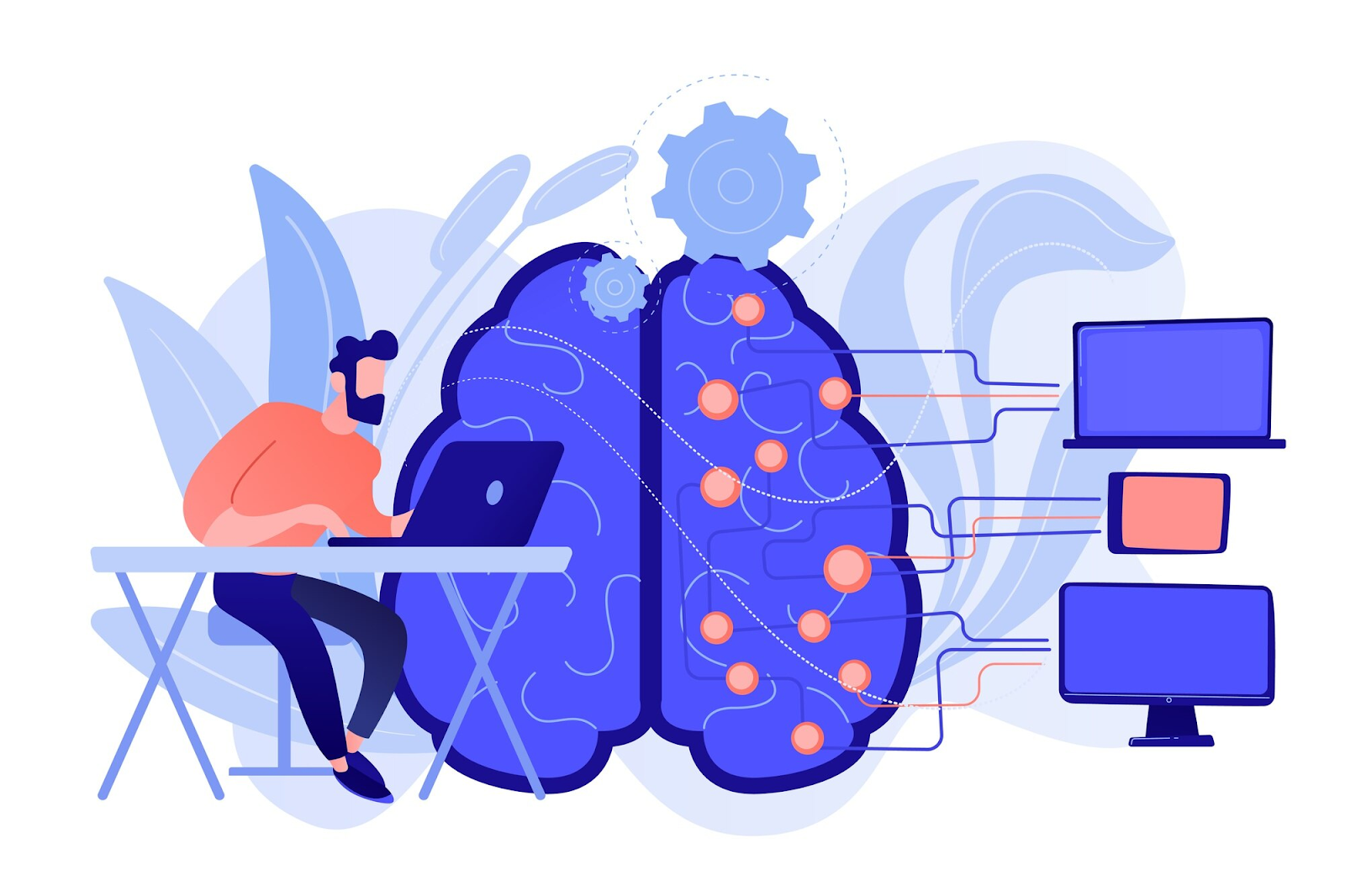
Source: Freepik
Theory of Mind in psychology refers to the ability to attribute mental states – beliefs, intents, desires, emotions, knowledge – to oneself and others, and to understand that others have minds with their own perspectives. When applied to AI, Theory of Mind AI would be an AI that can grasp the concept of mental states. In simple terms, this type of AI would be able to “understand” humans – including our emotions, motives, and intentions – and adjust its behavior accordingly.
This category of AI is still theoretical; no true Theory of Mind AI exists yet. It represents a future generation of AI that would need to go beyond cold calculation and incorporate social intelligence. For instance, a Theory of Mind AI interacting with a person could read the person’s facial expressions or tone of voice and infer their emotional state (“This customer is getting frustrated” or “my manager seems pleased with the results”), then modify its responses in a nuanced way (perhaps offering empathy or changing its explanation style).
Why is this important? Consider human teamwork and communication – so much of our interactions rely on understanding unspoken cues and emotions. An AI that lacks this will always be somewhat rigid or prone to miscommunication in roles that require human interaction. That’s why Theory of Mind AI is seen as a necessary step toward AI that can truly collaborate with humans in social or customer-facing environments.
Potential applications in business (if and when this AI becomes reality) include:
- Advanced customer service agents: Imagine an AI customer support representative that can detect a customer’s anger or confusion and adjust its approach – perhaps slowing down to patiently reassure an irritated caller, or injecting sympathy (“I’m sorry for the inconvenience, I can imagine that’s frustrating”) in a way that’s appropriate. It would be a game-changer for customer experience if AI could navigate emotional conversations smoothly.
- AI coaches or assistants: In workplace settings, a Theory of Mind AI assistant might gauge a team’s morale or a user’s stress level. For example, if an AI project management tool notices a team is overloaded (perhaps through sentiment analysis of communications), it could proactively suggest reallocating tasks or sending a gentle reminder to take breaks. It’s moving into the realm of EQ (emotional quotient), not just IQ.
- Negotiation and Sales AI: In wholesale trade or procurement negotiations, an AI agent with theory of mind capabilities might better interpret the counterpart’s subtle signals – are they hesitant about the price, are they eager to close quickly? – and adjust strategy (just as a skilled human salesperson or negotiator would).
Currently, research areas like affective computing (AI that can recognize and simulate emotions) and social robotics are the closest things to this. We see early elements, like AI that can recognize facial expressions or voice sentiment. Some customer service bots can do rudimentary sentiment analysis on text (happy vs angry customer) and escalate appropriately. But none of these truly understand emotion – they just classify it.
For AI to reach Theory of Mind, it would likely need multi-modal perception (vision, audio, perhaps biometrics) and sophisticated models of human psychology. It would need to handle the complexity that humans have different feelings and knowledge than the AI does itself. In essence, the AI would maintain an internal model like, “I know X, the human knows Y, the human feels Z, so I should act accordingly.” This is extraordinarily complex, but researchers believe it’s a crucial step toward more natural human-AI interaction.
From a decision-maker’s perspective, Theory of Mind AI is on the horizon, not here today. But its potential is exciting: it could make AI far more engaging and effective in collaborative roles. One could imagine future customer interaction AI in retail or hospitality that understands and responds to human emotions – providing a much more “human” touch than today’s chatbots. For example, a future AI concierge might sense a traveler’s stress after a long flight and proactively simplify the check-in process with a comforting tone and expedited steps, much like an empathetic human concierge would.
Experts often cite Theory of Mind AI as a bridge we must cross on the way to true AGI. If AGI is to interact with us as equals, it likely must have some theory of mind ability. However, building this raises not just technical questions but also ethical ones (e.g., should AI simulate empathy if it doesn’t actually feel anything? And will humans trust empathy from a machine?). Those are debates for the coming decades.
In summary, Theory of Mind AI:
- Is a future class of AI that would understand and respond to human mental states (emotions, intentions).
- Does not exist yet, but we see precursors in sentiment-aware AI and social robots.
- Could transform customer service, teamwork, and any AI-human interaction by adding emotional intelligence to machine intelligence.
For businesses, keeping an eye on this area is wise – especially industries like customer service, healthcare, or sales, where human empathy and understanding are central. Companies that plan ahead could eventually integrate Theory of Mind AI to elevate how their AI systems engage with people, giving them a competitive edge in user experience.
7. Self-Aware AI – AI with Consciousness (Hypothetical)
At the very end of the AI functionality spectrum lies Self-Aware AI. This is the hypothetical AI that not only understands others’ emotions and mental states (Theory of Mind) but also has a sense of self. In other words, a self-aware AI would have its own consciousness, feelings, and thoughts. It could say “I feel X” or “I want Y” and truly mean it, not just simulate it. This concept is essentially the AI achieving a level of awareness indistinguishable from human consciousness – or perhaps even alien to us if it’s a different kind of consciousness.
It must be stressed: Self-aware AI does not exist. It remains firmly in the realm of speculation and philosophical thought experiments. Some argue it may never be possible, while others think it could emerge as a byproduct if AI reaches and surpasses human intelligence (ASI). If an AI becomes superintelligent, one big question is whether it might also become self-aware (the two don’t necessarily have to coincide, but they could).
From a functional standpoint, a self-aware AI would have its own internal states and reflections. It would understand its own existence and perhaps have intrinsic motivations or goals. This raises some mind-bending scenarios. For instance, how would we ensure a self-aware AI’s goals align with ours? If it truly has desires, what if those conflict with human interests? This is why self-aware AI is often a topic in AI ethics and even science fiction storylines.
In practical business terms, it’s hard to even predict what self-aware AI would mean – it’s beyond AGI and ASI in some sense, because it’s not just about intelligence level but about the nature of the intelligence. Conceivably, a self-aware AI could make autonomous decisions not because it was programmed to optimize a metric, but because it “decided” it was the best course of action from its own perspective. For example, a self-aware supply chain AI might reconfigure your entire logistics network overnight because it believes that’s better – even if no one asked it to. This level of autonomy is both tantalizing (imagine an AI company CEO that runs the business 24/7 with superhuman acumen) and terrifying (the AI might pursue objectives that humans didn’t explicitly set).
At this point, discussing self-aware AI is largely theoretical and often overlaps with discussions about ASI. In effect, self-awareness would be a defining trait that could make an ASI truly independent of human oversight. It’s the kind of AI many movies portray – the machine that “wakes up” and starts making choices on its own. In reality, we are nowhere near this. Even detecting or defining consciousness in machines is an unsolved scientific question.
However, it’s useful for decision-makers to be aware of this concept, because it frames the ultimate ethical horizon of AI. If one day machines could have experiences, it might shift how we treat them (would a conscious AI have “rights”?). And leading AI researchers take these possibilities seriously when advocating for AI regulation and ethics today. The long-term societal impact of AI is considered significant enough that many experts call for proactive governance – not because your factory robot might demand civil rights tomorrow, but because instilling ethics in AI now is easier than trying to bolt it on later if AI grows more autonomous.
In summary, Self-Aware AI is the hypothetical stage where:
- AI achieves consciousness and self-recognition.
- It can understand its own internal state and potentially have its own desires or goals.
- This concept remains science fiction at present, but it represents the theoretical end-state of AI evolution in functionality terms.
For businesses today, self-aware AI doesn’t play into roadmaps (aside from maybe inspiring cool brainstorming). But it’s part of the larger narrative of AI development that responsible leaders keep in mind. It underscores why themes of AI ethics, control, and collaboration are recurring in industry forums. Even as we focus on practical Narrow AI projects, the question of “where is this all going eventually?” looms in the background. Savvy decision-makers will ensure their AI strategies are future-proofed and ethically grounded, so that as AI capabilities advance along these categories, their organizations can adapt and thrive alongside them.
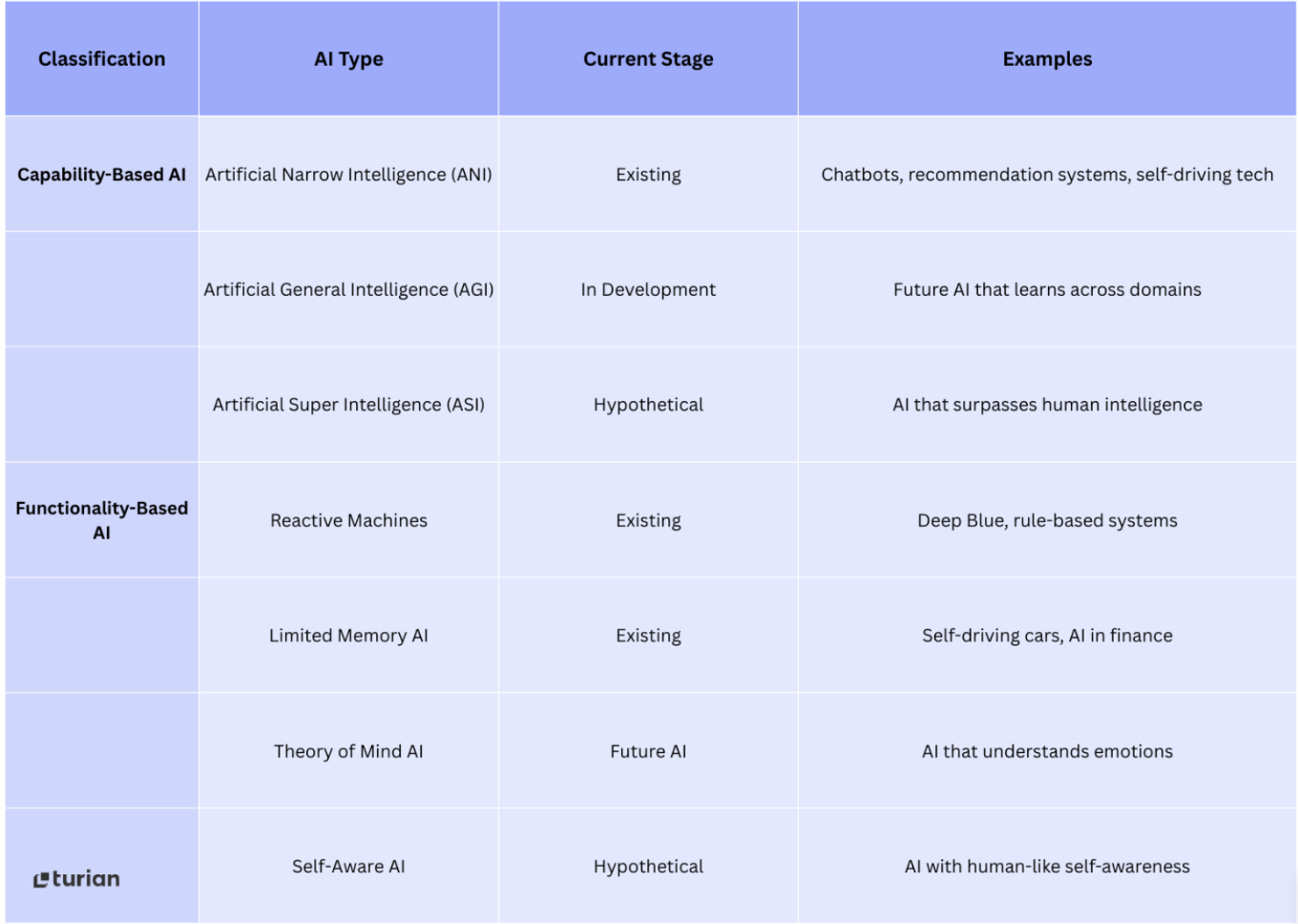
Source: turian
Implications and Balancing Theory with Business Reality
As we’ve seen, not all “types of AI” are equally present today – Reactive and Limited Memory AI are here now, while Theory of Mind and Self-Aware AI remain concepts for the future. Similarly, Narrow AI is ubiquitous now, General AI is on the horizon, and Superintelligence is speculative. Understanding these distinctions isn’t just academic; it has practical business implications:
- Strategic Adoption: Businesses can invest confidently in Narrow AI solutions (ANI) today – these are mature, with proven ROI in manufacturing, supply chain, and many other areas. Adopting machine learning for demand forecasting or using computer vision for quality control are low-regret moves that many competitors are already doing. According to IDC, by 2025 about 67% of AI spending will come from enterprises integrating AI into core operations – indicating that narrow AI will be deeply embedded in business processes. Organizations that fail to leverage these current AI types risk falling behind in efficiency and insight.
- Building Blocks for the Future: While AGI and advanced AI are not available to buy off the shelf, companies should start laying the groundwork. This means data infrastructure, talent, and AI governance. As an analogy, think of Narrow AI as the specialized tools and General AI as a potential super-employee; you want to have the right data environment and culture so that when more general AI tools emerge, you can onboard them seamlessly. Indeed, many leading companies are already experimenting with the most advanced ANI (like generative AI) to approximate some general capabilities, gaining experience with AI that can, for example, generate content or code across domains. A McKinsey’s survey shows an acceleration in AI adoption, and a focus on redesigning workflows to capture AI value. These are stepping stones toward more generalized AI usage.
- Risk Management and Ethics: The further out on the AI spectrum we go (AGI, ASI, self-aware), the more we encounter unknowns and risks. But even today’s AI can pose ethical challenges (bias in algorithms, lack of transparency, etc.). Businesses should implement responsible AI practices now, during the Narrow AI era, as a form of future-proofing. Frameworks for transparency, fairness, and human oversight will serve even better if/when AI becomes more autonomous. It’s a balancing act: capturing AI’s benefits while controlling its downsides. Addressing these issues early will make it easier to scale AI safely when more powerful types arrive.
- Human-AI Collaboration: With each step up in AI capability, the dynamics of human-AI interaction evolve. In the current state, humans firmly direct AI tools (e.g., a planner uses forecasting software). As AI gains more capability, the relationship may shift toward partnership – think of future factory floors where AI systems adjust processes on the fly and human managers act more like coordinators or exception handlers. Companies should foster a culture where AI is seen as a collaborator, not a threat. Training employees to work with AI (interpreting AI insights, checking AI outputs, and feeding the AI the right questions) is already important, given that 72% of firms use AI in at least one function. This will only grow in importance. Those who treat AI as “augmented intelligence” – a way to augment human decision-making – will extract the most value. According to PwC, nearly all business leaders are prioritizing AI initiatives in the near term, and part of that journey is figuring out how AI can best augment their teams.
- Industry Transformations: Certain sectors like manufacturing, wholesale, and logistics – the focus of our examples – are set to benefit immensely from even current AI types. These are industries with massive amounts of data and repetitive processes, ripe for Narrow AI optimization. As AI evolves, these sectors could be transformed by things like AGI (imagine a fully autonomous factory that manages itself end-to-end). But businesses don’t need to wait for that – there is a spectrum of “smart automation” technologies available now that progressively incorporate more AI. The competitive advantage comes from adopting the right AI type for the right problem.
72% of firms use AI in at least one function.
In balancing theory with reality, it helps to remember: AI is not a monolith. When someone asks “what are the different types of AI?” you now know to clarify – do we mean their general intelligence level or their functional design? This clarity helps in setting expectations. Narrow AI can do a lot today, but it won’t magically think like a human. Future AI might, but we must get there step by step.
Embracing AI’s Potential – A Call to Action

Source: Freepik
Artificial Intelligence spans a broad spectrum – from today’s simple, reactive machines and data-driven narrow AI systems, to tomorrow’s envisioned general intelligences and perhaps even self-aware machines. For decision-makers, understanding these main types of AI is more than an academic exercise; it’s a roadmap for innovation. It means knowing what AI can do now and what it might do soon, so you can invest wisely and strategically.
In practical terms, most businesses should be leveraging Narrow AI solutions (ANI) right away. These tools are battle-tested and can yield significant improvements in efficiency, cost, and insight. Whether it’s deploying machine learning for demand forecasting in your supply chain, using computer vision to enhance quality control in manufacturing, or automating routine customer inquiries with AI chatbots, Narrow AI is the here-and-now driver of ROI. The data and examples cited – from 20-30% inventory reductions in distribution to 10-15% productivity boosts in plants – show that AI is not hype; it’s happening. Enterprises that have embraced these AI types are outperforming peers and rewiring how they run.
At the same time, keep an eye on the horizon. Artificial General Intelligence, while not here yet, is being actively pursued by the world’s top AI labs. We see glimmers of its approach in advanced systems that appear to generalize (like GPT-style models). Staying informed about AGI’s progress – and even participating in pilot projects with the most advanced AI – can position your organization to “pounce” when the time is right. Just as importantly, engage with the ethical dialogue and frameworks around AI. This will ensure that as you scale AI, you do so responsibly, maintaining trust with customers and stakeholders.
For business leaders: the message is clear. AI is a game-changer in your fields, and it’s evolving fast. The capability-based and functionality-based lenses provided here equip you to ask the right questions when considering AI initiatives. Do we need a simple rule-based automation, or a learning system? Can a current AI solution handle this task, or are we bumping against a limitation that awaits a more general AI? Understanding the 7 types of AI gives you a conceptual toolkit to align business problems with the appropriate AI approaches.
Finally, consider partnerships to accelerate your AI journey. Very few organizations can do all of this alone. This is where turian’s AI capabilities come into play. turian specializes in cutting-edge AI solutions – from intelligent automation to advanced machine learning – and stays at the forefront of AI developments. We help bridge the gap between theory and practice, ensuring that our clients benefit from the latest AI innovations in a business-focused, ethical manner. Whether you’re looking to implement a narrow AI system to optimize part of your operation or laying the groundwork for more advanced AI down the road, turian can be your trusted partner in that evolution.
In an age where AI is swiftly becoming central to competitive strategy, the winners will be those who act – who pilot new technologies, scale what works, and continually educate themselves on the possibilities. The landscape of AI types will keep expanding, but one thing is constant: the need to leverage intelligence (human or artificial) to drive progress. Now is the time for action. Embrace the proven AI solutions available today, experiment with emerging AI trends, and cultivate an organization that is ready for whatever the next wave of AI brings.
As you plan your next steps, remember that every great AI achievement started with an informed decision to explore what’s possible. Let this understanding of AI’s types and potential be your springboard. It’s time to explore AI solutions for your business – and unlock new levels of performance and innovation with turian’s help. Together, we can navigate the AI journey from the present to the future, turning possibilities into tangible results.
{{cta-block-blog}}
Say hi to your
AI Assistant!

Lernen Sie Ihren KI-Assistenten kennen!
.avif)
FAQ
There are three broad categories of artificial intelligence (AI): Narrow AI, General AI, and Super AI. Narrow AI (or Weak AI) includes all the specialized AI and machine learning systems we use today that are designed for specific tasks – like virtual assistants or image recognition – and they excel at those tasks within their limited scope. By contrast, Artificial General Intelligence (AGI) is a theoretical form of AI with human-level cognitive abilities, capable of learning and understanding any intellectual task (something we haven’t achieved yet). Super AI is a hypothetical future level of intelligence that would far surpass human capabilities in all aspects, a concept currently found only in speculation and science fiction. This framework gives a simple way to understand the types of AI based on their overall capability (from narrow task-focused systems to imagined super-intelligences) and is a popular way to describe major artificial intelligence categories in tech discussions.
AI can also be classified by functionality into four types of AI: Reactive Machines, Limited Memory, Theory of Mind, and Self-Aware AI. Reactive machines are the most basic type, reacting to current inputs without any memory of past data (for example, a chess-playing AI that only evaluates the present board state). Limited Memory AI can utilize some past information or experience to inform current decisions, which is how many modern machine learning applications work – think of self-driving cars or recommendation systems that learn from recent data. Theory of Mind AI refers to a still-theoretical concept of AI that could understand human emotions, beliefs, and intentions, enabling more natural and adaptive interactions. Finally, Self-Aware AI is the speculative idea of an AI that has its own consciousness and self-awareness – these last two types are only hypothetical at this stage and do not exist in practice. This functional classification shows how artificial intelligence can range from simple reactive systems to the imagined self-aware machines of the future, helping beginners grasp the scope and progression of AI development.
Narrow AI and General AI are very different categories of intelligence in machines. Here are the key differences between weak AI vs. strong AI:
- Scope: Narrow AI (weak AI) is specialized to perform a single task or a limited set of related tasks. General AI (strong AI) would have a broad scope, meaning it could handle any task or problem, much like a human can.
- Flexibility: A Narrow AI system cannot learn or operate outside its specific domain – it does one thing really well and nothing else. A General AI would be highly flexible, able to learn and adapt to new tasks and unfamiliar situations on its own.
- Current Status: Narrow AI exists today in many forms (e.g., voice assistants, game-playing AIs, recommendation systems). In contrast, General AI does not exist yet – it’s a goal for the future. No present-day AI has the generalized understanding and learning capability that would qualify as AGI.
In summary, narrow AI is task-specific and present in today’s technology, whereas general AI would be human-level versatile intelligence and remains theoretical.
No – we have not achieved general AI or super AI at this time. All current AI systems are forms of narrow AI, meaning they operate within predefined domains and tasks. There is no AI yet that possesses human-level general intelligence across diverse tasks (AGI), and certainly no superintelligent AI that exceeds human abilities. Researchers and scientists are actively working toward Artificial General Intelligence, but it remains a long-term goal and an unsolved challenge. Super AI is even more speculative – it’s a concept for the far future. As of today, when you interact with an "AI" in the real world, you’re interacting with a narrow AI tailored for a specific purpose, not a general or super-intelligent being.