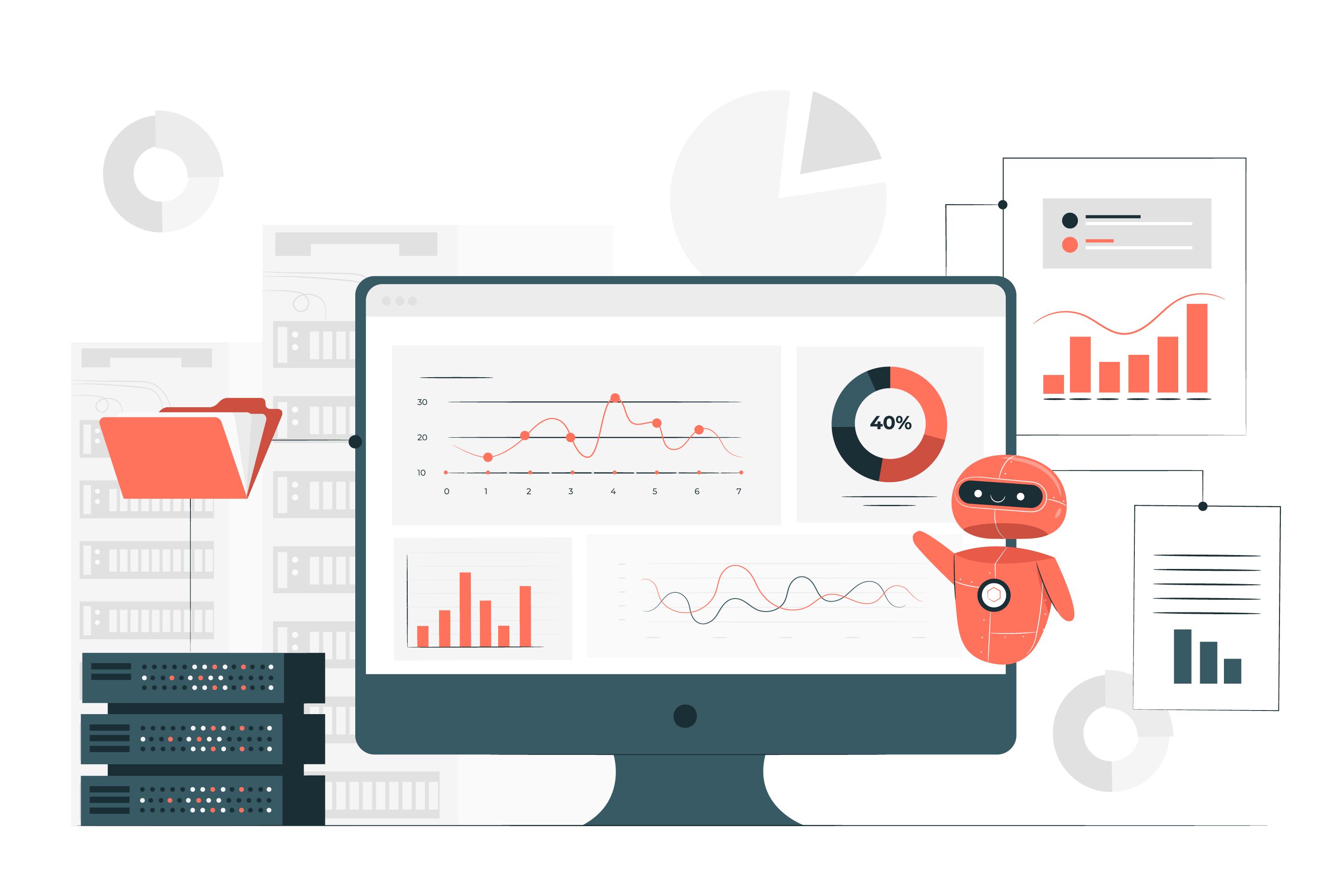

7 AI Trends to Watch in 2025: A Guide for Businesses
Artificial Intelligence (AI) is evolving at breakneck speed and becoming a cornerstone of modern business. From small startups to global enterprises, organizations are leveraging AI to automate workflows, augment decision-making, and gain competitive advantage. In fact, about 72% of organizations have now adopted some form of AI, up from roughly half just a few years ago. These AI industry trends aren’t confined to tech giants or mid-tier firms; they’re reshaping operations and strategy for businesses of all sizes and sectors – even SMBs are joining the AI revolution – 75% of small and mid-sized businesses are already experimenting with AI (rising to 83% among high-growth SMBs). The use of cutting-edge tools like generative AI is also surging – 65% of companies were using generative AI in 2024, nearly double the share in 2023. These figures underscore that AI is not hype; it’s a lasting shift in how businesses operate.
Why does this matter going into 2025? Because we’re reaching a point where AI is moving from experimentation to execution. IDC forecasts worldwide spending on AI technologies will exceed $600 billion in 2028 as organizations embed AI across their operations. This wave of investment is driven by tangible results – according to EY, a near-unanimous 97% of companies already investing in AI report positive ROI on those projects. The message is clear: AI is delivering real business value, and executives are responding by doubling down.
What are the key AI trends for 2025 that every business should know? Below, we break down the top 7 AI trends shaping the landscape – from autonomous AI agents and hyperautomation to edge computing and governance. Each trend is not just a tech buzzword but a strategic insight that decision-makers can use to future-proof their business. Let’s dive into the AI trends to watch in 2025 that can drive innovation and operational excellence in your organization.
AI Trend 1: Meet Your New Digital Colleague – The Rise of AI Agents

Source: Freepik
One of the most talked-about trends in 2025 is the rise of AI agents – intelligent assistants or “virtual co-workers” that can autonomously perform tasks and make decisions. Advances in generative AI and reinforcement learning have enabled agents that don’t just respond to commands, but proactively execute multi-step processes. Gartner has named “Agentic AI” a top strategic technology trend for 2025. These agents can monitor systems, analyze data, and take actions without human intervention. Entry-level use cases involve offloading routine work (like scheduling or basic customer inquiries), while more advanced agents manage complex operations and even mentor employees.
The impact on productivity could be enormous. Gartner predicts that by 2028, at least 15% of all daily work decisions will be made autonomously by AI agents – up from less than 1% in 2024. Likewise, the number of enterprise software applications featuring built-in AI agents will surge from less than 1% in 2024 to 33% by 2028. Early adopters across industries are already seeing benefits – such agents act as tireless coworkers that work 24/7, helping businesses respond faster and free human employees for higher-value work.

Source: Created by turian, based on data from Gartner (2024).
So what sets AI agents apart from the automation we’ve seen before? Complexity and autonomy. Traditional automation (including many chatbots or RPA scripts) follows pre-defined rules and workflows – if X then Y. AI agents, on the other hand, use AI models to perceive and interpret information, then plan and execute tasks with minimal oversight. They can make judgment calls within set parameters. For example, in IT operations, an AI agent might monitor system performance, detect an anomaly, and proactively apply a fix. These agents are essentially digital employees: they can be assigned objectives and will carry them out, learning and adapting as they go. This goes beyond a chatbot that just answers questions – the agent can take real actions through software integrations.
AI agents are transforming work in sectors from finance (automating compliance checks) to manufacturing (intelligent supply chain monitoring) and retail (virtual sales assistants). Not just for large enterprises, they are accessible to smaller firms via cloud-based AI services. With tech giants releasing AI copilots and startups launching specialized agents, businesses of all sizes can deploy virtual assistants for tasks like data entry, IT support, or employee training. Of course, this trend raises workforce questions – there’s excitement about productivity, but also fears of job displacement. It’s important to note that AI agents are not omnipotent – they excel in bounded tasks with clear goals and lots of data, but still require oversight for complex or sensitive decisions. Companies are finding that the best results come when AI agents and humans collaborate, each focusing on what they do best. Moving into 2025, savvy organizations will pilot AI agents as “virtual team members” to boost efficiency while retraining staff for more strategic roles.
AI Trend 2: Hyperautomation Hits Its Stride
If 2020-2023 were about adopting automation for individual tasks, 2025 is about automating entire processes – enter hyperautomation. Hyperautomation refers to the coordinated use of multiple technologies – AI, machine learning, robotic process automation (RPA), workflow tools, and more – to rapidly identify, vet, and automate as many business processes as possible. It’s an end-to-end approach. Rather than just deploying a bot to input data from one system to another, companies are now stringing together automation solutions so that an entire workflow (say, order-to-cash or employee onboarding) can run with minimal human intervention. Gartner calls hyperautomation a top trend in automation, forecasting that by 2025 hyperautomation will impact one-fifth of all business processes. The promise is huge: higher efficiency, lower costs, fewer errors, and the ability to scale operations quickly.
What does hyperautomation look like in practice? Consider a financial workflow like invoice processing. In a hyperautomated scenario, an incoming invoice PDF might be automatically read by an AI OCR (optical character recognition) engine, key details extracted and entered into an accounts payable system by an RPA bot, then an AI algorithm checks the invoice against purchase orders for accuracy and compliance. If everything matches, the system automatically approves payment; if not, it routes to a human for review. No more paper shuffling. Or take contract review in legal or procurement: AI can now scan lengthy contracts, flag important clauses or deviations, and even suggest edits. A process that took days of human review can be shortened to minutes with AI assistance, with lawyers only focusing on the truly novel or risky portions. By chaining these AI-driven steps together, businesses automate not just tasks but decisions – for example, an insurance company might automate claims handling up to a certain dollar amount using AI rules and only involve human adjusters for larger or complex claims.
Crucially, hyperautomation isn’t just about efficiency – it’s about redeploying human talent to higher-value activities. When routine work is automated, employees can focus on strategy, creativity, and relationships. Early adopters of hyperautomation report significant benefits. Many firms see major operational cost reductions – one survey found 59% of organizations implementing RPA/AI at scale saw cost savings and over 86% saw productivity gains. Hyperautomation can also speed up processes dramatically (cycle times cut from days to hours) and improve accuracy (fewer manual errors). This directly impacts the bottom line – faster processes mean faster sales cycles and happier customers, and fewer errors mean better compliance and quality.
In 2025, we expect hyperautomation to hit its stride because the enabling technologies have matured and become more accessible. Low-code platforms (see Trend 3) let business analysts themselves map out and deploy automated workflows without heavy IT lift.
The DACH region’s enterprises, known for focus on operational excellence (e.g., Germany’s manufacturing sector), are especially keen on hyperautomation as a way to bolster productivity amid skilled labor shortages. However, a challenge remains: not every process should be automated, and not every automation will yield ROI. Successful hyperautomation requires careful selection of targets (prioritize high-volume, repetitive, rule-based processes), change management, and ongoing maintenance. Nonetheless, as the tools improve and success stories pile up, 2025 will see hyperautomation move from pilot projects to broad implementation across business units. In short, automation is no longer a piecemeal effort – it’s scaling company-wide.
AI Trend 3: Democratizing AI – Low-Code and No-Code Solutions

Source: Freepik
One of the most exciting trends in automation and AI is that it’s becoming far more accessible. The rise of low-code and no-code AI platforms means that you don’t need a PhD in machine learning – or even a large software development team – to implement AI solutions in your business. Low-code/no-code tools provide visual interfaces, drag-and-drop modules, and pre-built AI components that let users build applications or workflows with minimal hand-written code.
In 2025, this democratization of AI is accelerating, enabling “citizen developers” (business users with domain knowledge but limited coding skills) to create AI-driven apps and automations. Low-code AI platforms are at the heart of this trend. They provide visual interfaces and modular components (think Lego blocks of AI functions) to assemble intelligent applications quickly. Gartner projects that by 2025, 70% of new enterprise applications will be developed using low-code or no-code technologies, up from less than 25% in 2020. In the AI space, that means many business processes will get an AI infusion without a heavy lift on the IT side.
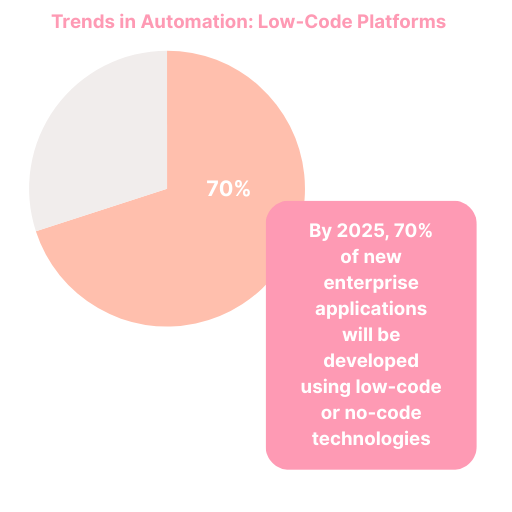
Source: Source: Created by turian, based on data from Gartner (2021).
Why is low-code AI a game-changer for businesses? First, it slashes development time and cost. Instead of waiting months for a custom AI software project, a business analyst can use a no-code interface to set up, say, a predictive model that forecasts inventory needs, or an AI chatbot to handle support queries, within days or weeks. Companies can experiment more and iterate quickly.
Secondly, low-code platforms spread the load by empowering existing staff to implement solutions. For example, a marketing manager could use a low-code tool to build a lead-scoring model that ranks sales leads by quality using an embedded machine learning algorithm – without having to write the algorithm from scratch. Or an operations manager could automate approval workflows with AI checks (like automatically flagging unusual orders) via a visual workflow builder. This self-service AI development unlocks innovation at the edges of the organization
The capabilities of low-code/no-code AI have expanded greatly. Many RPA vendors now offer AI modules (for reading documents, classifying data, etc.) that non-programmers can insert into automation sequences. Cloud providers like Microsoft and Google have low-code AI builder studios where you can train models on your data with a few clicks. There are no-code chatbot builders that let you create a conversational AI by just providing examples of Q&A. Even more advanced uses – like analyzing datasets for trends or creating dashboards with embedded AI insights – have become easier with tools that auto-generate code or queries based on plain language instructions.
Of course, democratizing AI raises questions of governance – if everyone can build an AI workflow, how do you ensure they all comply with data security and ethical guidelines? Leading organizations address this by establishing guardrails and offering training for citizen developers. IT departments shift to a role of enablers and governors, setting up secure low-code environments and reviewing apps for risks. Overall, 2025 will see AI truly in the hands of the many, not just the few. Businesses that embrace low-code AI will be able to innovate faster and involve more of their team in digital transformation, which is a competitive advantage in its own right.
AI Trend 4: Further Advances in LLMs (Large Language Models)
Large Language Models (LLMs) – the AI models behind GPT-4, ChatGPT, Bard, and other generative AI – took the world by storm starting in 2023. In 2025, LLMs are becoming even more powerful, versatile, and integrated into business operations. We’re moving beyond the novelty of AI writing a poem or answering trivia; the next generation of LLMs is tackling real enterprise challenges: analyzing complex documents, drafting business content, powering customer service agents, and even performing advanced reasoning tasks. Model architectures are improving (with techniques to make responses more accurate and grounded), and companies are increasingly able to customize these models with their own data.
One key advancement is that LLMs are getting better at understanding context and specialized knowledge. Where earlier models might falter on domain-specific questions (e.g., medical or legal details), newer models trained with plugin architectures or fine-tuned on industry data can perform far more reliably.
From a business use-case perspective, customer service is a prime beneficiary of better LLMs. Instead of simple chatbots with scripted answers, companies are implementing AI chat agents powered by LLMs that can hold more natural and nuanced conversations. These AI agents can understand a customer’s free-form question, access the right information (like checking an account or knowledge base), and generate a helpful response – often indistinguishable from a human representative. This greatly improves customer experience and scalability of support. Automated content creation is another big one: marketing teams use LLMs to draft social media posts, product descriptions, or even first drafts of articles, which humans then refine. This speeds up content pipelines significantly. Contract analysis and legal drafting have also been transformed – law firms and legal departments use LLM-based tools to review contracts, highlight risks, and even suggest language, cutting down legal review times. Many employees have effectively gotten an AI co-pilot: salespeople use AI to generate personalized outreach emails, data analysts use AI to write code or formulas, and HR uses AI to write policy drafts or job descriptions. These scenarios were experimental a year or two ago; now they’re becoming standard practice.
Adoption statistics show LLMs are rapidly entering the enterprise mainstream. McKinsey’s latest AI survey found that by 2024, one-third of organizations had integrated generative AI (like LLMs) into at least one function, and this number is climbing fast.
AI Trend 5: Humans + AI – The Rise of the Augmented Workforce
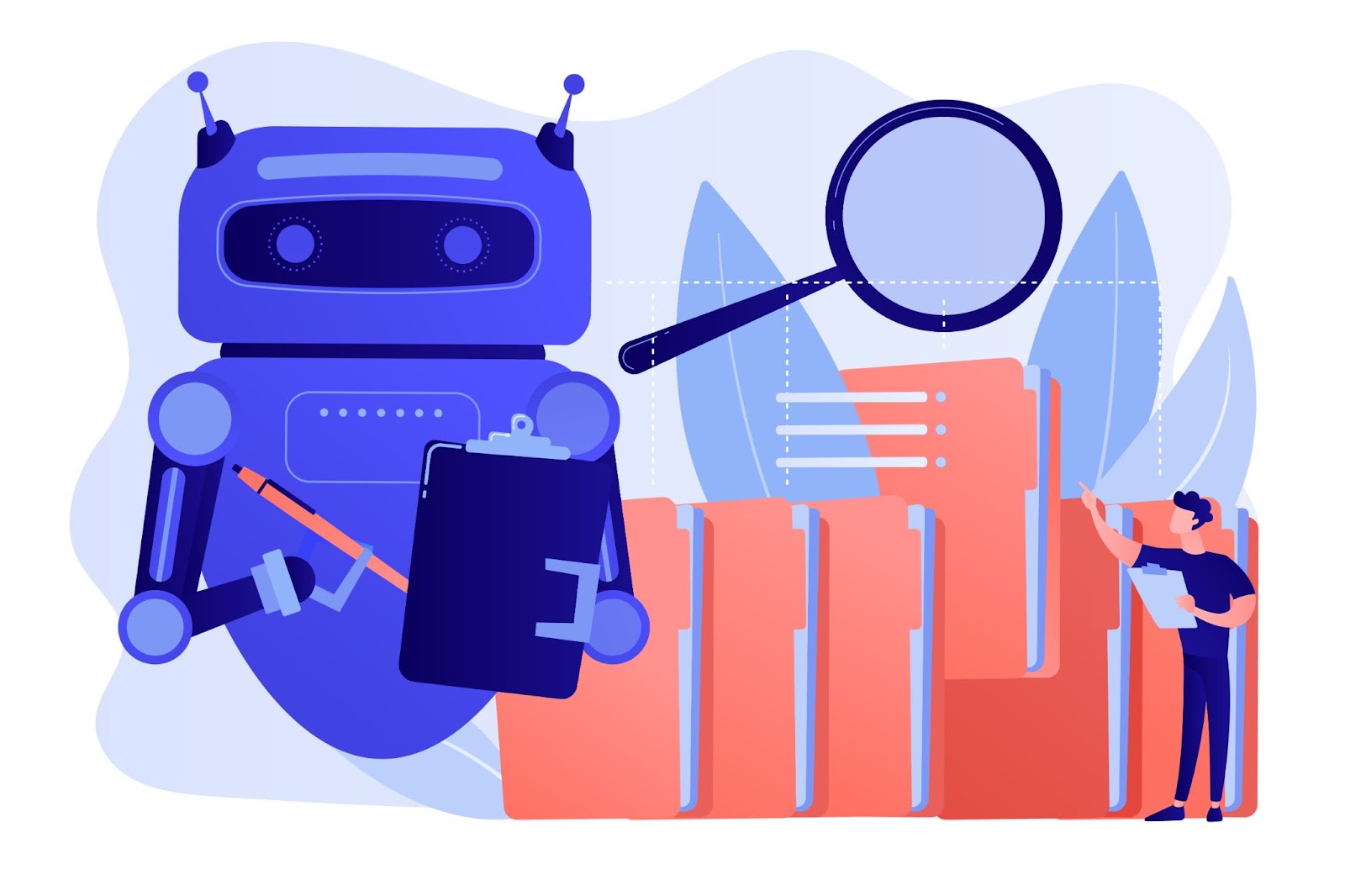
Source: Freepik
Rather than AI replacing humans, the narrative for 2025 is very much about AI augmenting the workforce. The concept of an “augmented workforce” means employees armed with AI tools, leading to a collaboration where human strengths (creativity, judgment, empathy) combine with AI strengths (speed, accuracy, scale) for superior outcomes. In practice, augmentation is showing up everywhere: a salesperson uses an AI assistant to get meeting summaries and next-step suggestions after sales calls; a doctor uses AI to analyze medical images, aiding their diagnosis; a supply chain manager relies on AI forecasts to decide stock levels. Far from making people obsolete, these AI tools are helping people work smarter and focus on higher-value tasks. As a result, companies are seeing substantial productivity gains and employees are reporting higher effectiveness when working with AI. A 2025 executive survey illustrates this, with 58% of business leaders reporting exponential productivity or efficiency gains from generative AI tools, and another 16% saying these tools have freed staff from repetitive tasks.
A 2025 survey of executives found that a majority see significant productivity improvements from AI. 58% report “exponential” efficiency gains, and another 16% say AI has freed workers from routine tasks – highlighting the impact of an augmented workforce.
Instead of viewing AI as a threat to jobs, many forward-looking organizations see it as a performance booster for their teams. With AI helpers, each employee’s capacity can increase. A common refrain is that AI won’t replace you, but “an employee who knows how to use AI might.” This underscores why training and upskilling employees to work alongside AI is a top priority. In fact, companies are rolling out training programs to raise AI literacy – teaching staff how to interpret AI outputs, how to ask the right questions (prompts), and how to integrate AI into their daily workflows. According to Gartner, by 2025, at least 50% of organizations will have some form of AI skills training in place for their workforce (and this percentage is likely even higher in tech-forward regions).
Let’s quantify the benefits: A survey of 400 office workers found 61% reported that AI tools enhanced their efficiency and productivity in their jobs.
AI Trend 6: Edge AI and IoT: AI on the Edge
As AI models become more efficient and the Internet of Things (IoT) continues to explode, a significant 2025 trend is the shift of AI from the cloud to the edge. Edge AI refers to running AI algorithms locally on devices (or nearby on local servers) rather than in a centralized cloud data center. This is crucial for use cases that require real-time processing, low latency, enhanced privacy, or operation in bandwidth-constrained environments. Think of a factory machine that can instantly detect a defect on the production line using an onboard vision AI chip, or a smartphone that can translate speech to another language on the fly without sending data to the cloud. With billions of IoT sensors and devices generating data, it’s increasingly efficient to process data at or near the source. In fact, IDC forecasts that by 2025 there will be 55.7 billion connected IoT devices and sensors online globally – a staggering amount. Handling the data deluge from these devices requires distributing intelligence outwards, closer to where data is created.
The growth of edge computing infrastructure and 5G networks is propelling this trend. Faster local networks and specialized hardware (like NVIDIA’s edge GPUs, Google’s Coral AI edge tensor processors, etc.) enable devices from cameras to cars to run fairly advanced AI models. The result is “smart” everything – smart cameras, smart appliances, smart vehicles – that can analyze situations and make decisions instantly. For businesses, edge AI can improve responsiveness (e.g., an autonomous drone can avoid obstacles without a cloud round-trip), reduce cloud costs by filtering data (sending only important events upstream), and enhance reliability (critical systems can keep working even if connectivity drops). It also can address data sovereignty and privacy concerns, since sensitive data can be processed on-site without transmitting personal information to external servers.
Edge AI is particularly impactful in industries with physical operations. In manufacturing and energy, edge AI systems monitor equipment and detect anomalies or safety hazards in milliseconds, preventing failures and downtime. Retailers use edge AI in stores for applications like smart shelving (sensors that track inventory and shopper behavior without constant cloud reliance) and checkout-free payment systems. Healthcare is leveraging edge AI in medical devices – for example, wearable health monitors that analyze vital signs on-device to alert patients of irregularities immediately. Automotive is a huge driver: autonomous vehicles and advanced driver-assistance systems depend on edge AI to recognize objects, pedestrians, and driving conditions in real time for split-second decisions.
Additionally, edge AI opens up AI benefits to remote locations (mines, offshore oil rigs, rural farms) and reduces dependency on always-on internet. For smaller businesses, edge AI can be as simple as a security camera with built-in AI that flags suspicious activity (no expensive cloud video analytics service needed).
The adoption of edge AI often correlates with infrastructure maturity. Regions like North America, Europe, and East Asia (Japan, South Korea, China) with widespread 5G and advanced semiconductor industries are leading in edge AI deployments. For instance, smart city projects in Asia use edge AI for traffic management (cameras/processors at intersections optimizing flow locally).
In Europe, strict data protection rules encourage processing data on-site – companies there see edge AI as a way to comply with GDPR by keeping personal data in local devices or on-premise. This is especially relevant for sectors like healthcare or finance in the EU, where transmitting raw data to the cloud can raise legal issues; edge AI offers a compliant alternative by anonymizing or aggregating data at the source.
In developing regions, edge AI could leapfrog limitations in connectivity – local AI processing can enable functionality even when internet access is intermittent or slow. However, cost can be a factor: edge devices with AI capabilities are pricier, so large-scale deployment may come slower in cost-sensitive markets until economies of scale drive device prices down.
Globally, another consideration is interoperability and standards. As billions of edge AI devices roll out, there is a push (by organizations like the OpenAIOT consortium) for common standards to ensure these devices can communicate and be managed securely across regions. Additionally, countries are starting to consider regulations specific to edge devices (for security, given the risk of many “smart” endpoints being hacked). In 2025, expect to see more cross-border collaboration on securing IoT and edge AI systems, since data and cyber threats don’t respect geographic boundaries.
AI Trend 7: AI Governance and Responsible AI Frameworks – Building Trust in AI Initiatives
As AI adoption skyrockets, companies are realizing that robust governance, ethical safeguards, and compliance are not optional – they’re essential. Thus, a major trend for 2025 is the focus on AI guardrails and governance frameworks to ensure AI is used responsibly and transparently. Executives now rank AI-related risks (like data privacy, bias, and security) among their top concerns when deploying AI.
Organizations are establishing formal AI governance bodies, policies, and tools to keep their AI initiatives in check. In McKinsey’s 2025 AI survey, 28% of respondents from companies using AI said their CEO is now directly responsible for AI governance, and 17% have board-level oversight – indicating how seriously this is being taken at the top.
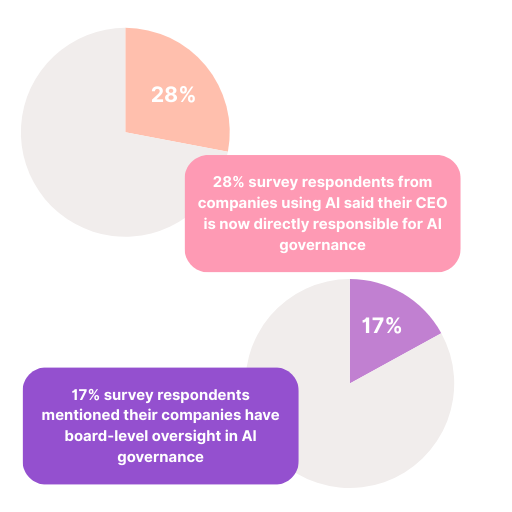
Source: Source: Created by turian, based on data from McKinsey (2025).
What do these guardrails look like? At a high level, businesses are implementing Responsible AI principles – guidelines that might include fairness (preventing bias or discrimination by AI), accountability (clear ownership of AI outcomes), transparency (explainability of AI decisions), and security/privacy (protecting data and ensuring AI can’t be easily abused). Concretely, companies are conducting AI risk assessments before deploying an AI system. For example, a bank introducing an AI loan approval model will assess if the model has bias against any demographic group and will put in monitoring to catch any drift in behavior. Many are adopting tools for AI explainability, so that when an AI makes an important decision, there’s a way to interpret why (this is important not just for trust, but sometimes for regulatory compliance). Businesses are also setting up processes like human-in-the-loop checkpoints – ensuring a human reviews AI outputs in cases that are sensitive or high impact. In customer service chatbots, if the AI is unsure or the customer is getting frustrated, it routes to a human. In hiring, if AI filters candidates, a human recruiter might double-check borderline cases to avoid missing great talent due to a quirk of the algorithm.
One major driver of this trend is external regulation. The EU AI Act is a landmark piece of legislation making its way into law, set to be one of the first comprehensive legal frameworks for AI. The AI Act will classify AI systems by risk levels (unacceptable risk, high risk, limited risk, minimal risk) and impose requirements accordingly. In parallel, the EU is also updating liability laws – proposing an AI Liability Directive and revising product liability rules to cover damage caused by AI-driven systems. This means businesses could be held accountable for AI decisions in new ways, raising the stakes for getting governance right. Europe’s proactive stance has inspired many companies globally to preemptively adopt similar standards.
The private sector is also responding with tools: 2025 will see wider use of AI governance platforms – software solutions that help track and enforce AI policies. These platforms can automate checks for bias in models, maintain model inventories, log AI decisions, and ensure overall legal, ethical, and operational compliance.
As we move through 2025, expect AI audits and certifications to become commonplace. Just like financial audits, AI systems (especially those outward-facing or critical) will go through periodic reviews. There’s talk of certifications or seals for AI ethics compliance that companies can use to signal to customers that their AI is trustworthy. The end goal of all this is to ensure that as we embrace AI more deeply, we don’t lose the trust of customers, employees, or society. Businesses know that AI’s benefits come with responsibilities. Those that navigate this well – building strong guardrails while still innovating – will enjoy the rewards of AI with far fewer downsides. Trustworthy AI is good for business.
Preparing for the AI-Powered Future: How Companies Can Stay Ahead
AI is no longer a moonshot; it’s a business reality. The trends discussed paint a picture of a future where AI is embedded in almost every aspect of business. To thrive in this future, companies need to be proactive and strategic in how they adopt AI. Here’s a 3-step roadmap for businesses to adopt AI responsibly and effectively:
- Assess AI-Readiness and Identify High-Impact Areas: Start by evaluating where your organization stands on the AI maturity curve. Do you have the necessary data infrastructure? Are your employees open to new tech? Do your teams have basic AI literacy? Identify gaps in skills or technology that need addressing (for example, you might need to modernize your data storage or hire a data scientist). Simultaneously, look for the high-impact use cases in your business. Not every process will benefit equally from AI – prioritize areas with lots of data, repetitive tasks, or clear pain points. The goal of this step is to create an AI opportunity map aligned with your business strategy, and to ensure you have a realistic understanding of what it will take (infrastructure, talent, change management) to implement AI solutions.
- Choose Scalable Solutions to Start Small and Expand Gradually: It’s wise to start with pilot projects, but choose technologies and partners with an eye toward scalability. For instance, if you’re implementing a chatbot, pick a platform that can later be expanded to other channels or languages if the pilot succeeds. Keep pilots focused: define success metrics (KPIs) in advance, such as “reduce processing time by 30%” or “increase sales conversions by 5% with AI recommendations”. This will allow you to evaluate ROI objectively. When a pilot hits its targets, champion its expansion. On the flip side, if an AI trial underperforms, analyze why (was the data insufficient? users not trained? etc.), adjust and iterate. Modern AI platforms (from cloud AI services to RPA suites) often allow modular scaling – you can add more processes or users to the existing setup rather than starting from scratch each time. Also, consider partnering with experienced vendors or consultants for initial implementations – they can bring expertise and frameworks.
- Train Employees to Collaborate Effectively with AI: The best technology deployment can falter if people don’t use it or use it incorrectly. Investing in your human capital to complement AI is essential. This means training employees at all levels – not just the IT folks – about what AI is, how it works, and how to work alongside it. In addition to tool-specific training, consider broader upskilling: for instance, teach staff how to frame good questions to AI (prompt engineering basics), or how to validate AI outputs. This builds a workforce that is AI-literate and can extract maximum value from the tools. Also, clarify roles – help employees understand that AI is there to assist, not judge or replace them. This alleviates anxiety and fosters adoption.
By following these steps – assess and target, start smart and scale, and empower your people – businesses can adopt AI in a way that is both responsible and strategically sound. It’s also wise to leverage external solutions where it makes sense instead of reinventing the wheel. This is where turian’s AI-powered solutions come into play. turian offers a suite of AI and automation tools that can help companies streamline workflows, enhance decision-making, and improve efficiency across various functions. Whether it’s an AI agent for your customer service, a hyperautomation platform for your operations, or analytics tools that unlock insights from your data, turian’s solutions are designed to be scalable and enterprise-ready, with built-in governance features. They enable businesses to implement advanced AI capabilities quickly (“start small”) and expand as needed (“scale gradually”), without compromising on compliance or requiring massive in-house development. By partnering with an expert provider like turian, companies can accelerate their AI journey – getting access to proven technology and support, and focusing their energy on using AI, not building it from scratch.
In conclusion, the year 2025 is set to be a transformative one for businesses that embrace these AI trends. The technologies and practices discussed in this paper show that AI is not just an IT project, but a strategic imperative touching every part of an organization.
By understanding the top AI industry trends and taking proactive steps to leverage them, companies can future-proof their business in the truest sense. The competitive gap between AI leaders and laggards will continue to widen. Those who act now to invest in skills, infrastructure, and the right partnerships will be the ones reaping the rewards in the years to come.
Ready to future-proof your business with AI? Explore how turian’s AI solutions can help streamline your operations and drive growth. Whether you’re just starting out or looking to scale up your AI initiatives, turian can provide the guidance and solutions to ensure you stay ahead of the curve. Book a demo today to unlock the power of AI in your enterprise and embark on the journey toward a smarter, more efficient future.
{{cta-block-blog}}
Say hi to your
AI Assistant!

Lernen Sie Ihren KI-Assistenten kennen!
.avif)
FAQ
In 2025, seven major AI industry trends stand out for businesses:
- AI agents will act as autonomous digital colleagues that take on tasks and make decisions.
- Hyperautomation will integrate AI, machine learning, and RPA to automate entire workflows.
- Low-code and no-code platforms will let non-programmers build AI solutions.
- Large Language Models (LLMs) will be used for more advanced tasks like document analysis and generation.
- AI will augment the workforce, boosting productivity rather than replacing roles.
- Companies will increasingly focus on AI projects with proven ROI.
- Governance and ethics frameworks, like the EU AI Act, will become mandatory for responsible deployment.
Hyperautomation expands beyond traditional task-level automation by combining technologies like AI, RPA, machine learning, and process mining to automate complex, end-to-end workflows. Instead of scripting a single task, hyperautomation creates interconnected, adaptive processes that can handle decision points, unstructured data, and real-time changes. It’s not static but continuously evolves by identifying new optimization opportunities. This allows companies to automate entire business operations, from order intake to shipping, with minimal manual input.
The dominant view for 2025 is that AI will mostly augment the workforce. While some roles focused on repetitive tasks may shrink, most jobs will evolve. AI is being used to handle routine work, freeing employees to focus on strategy, creativity, and people-centered tasks. Surveys show that workers generally feel more productive with AI tools. New roles such as AI trainers and prompt engineers are emerging, and companies that invest in upskilling are better positioned to benefit. The net effect of AI is expected to be job transformation and productivity gain, rather than widespread job loss.
The EU AI Act introduces a risk-based regulatory framework for AI, with strict requirements for high-risk systems and outright bans for unacceptable uses like social scoring. For companies in Germany, Austria, and Switzerland, this means preparing by identifying high-risk applications, ensuring transparency, oversight, and updating models to comply with ethical and legal standards. The Act starts applying in phases from 2025 and becomes fully enforceable by 2026. Like the GDPR, it carries large penalties for violations and will require businesses to integrate compliance into their AI development process.
Companies should start with focused pilot projects that have high impact potential and measurable outcomes. Clean, accessible data is essential, as is having the right platform or tools. Once a proof of concept is built, results should be compared against a baseline to demonstrate business value. User adoption is key, so training and clear communication are important. Successful pilots should then be scaled gradually and aligned with company strategy. Tracking ROI means monitoring both costs (development, infrastructure) and gains (time saved, revenue increase). Many firms bring in expert vendors to speed up deployment and reduce risk. With the right approach, AI implementation becomes a cycle of experimentation, learning, and scaling—maximizing both impact and returns.